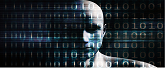 |
In a recent interview with Supply Chain Management Review, A.T. Kearney Partner Michael Hu discussed AI's impact on supply chain planning.Artificial Intelligence & Machine LearningArtificial intelligence (A.I.) and machine learning (ML) as a technology enabler can help address the fundamental structural challenges facing end to end planning today: faster and better demand prediction based on disparate data; automating mundane and labor-intensive activities; real-time decision twins to drive trade-offs; and enabling a leaner more integrative planning organization, to name just a few. Many organizations today are starting to pilot and experiment with A.I./ML across their planning organization – often in point solution mode such as using ML for predictive demand forecasting or using RPA to automate mundane workflow tasks. However A.I./ML by itself is not sufficient – supply chain organizations need to go from simply digitizing existing processes and workflow to reinventing the supply chain planning model to enable more flexible “sensing and pivoting”. For example, how would S&OP activate daily or even hourly for cross-functional decision trade-offs? What are the new feedback loops between production planning to demand forecasting? etc. In the Supply Chain Management Review Webcast "AI and Supply Chain Planning" (watch video to the right) we discussed several steps in getting started – including articulating a future vision of what S&OP model looks like, starting to get a handle on integrating disparate data source for training and scaling A.I./ML solutions and developing a fail fast MvP (minimum viable product) mentality. The Webcast featured six key takeaways: - Businesses across all sectors are pressuring supply chains to become a competitive weapon - to be a batch of one, as you want it, when you want it, where you want it.
- This pressure leads to major structural headwinds for planning and S&OP. Companies will increasingly be caught in a “performance trap,” throwing ever more headcount and investment just to stay in the same place in terms of service, inventory cost, and speed.
- AI and machine learning can serve as technology enablers to address the fundamental structural challenges facing end-to-end planning today: faster and better demand prediction based on disparate data; the automation of mundane and labor-intensive activities; real-time decision making to drive trade-offs; and the enablement of a leaner, more integrative planning organization, to name just a few.
- Many organizations today are piloting and experimenting with AI and machine learning across their planning organization - for example, using machine learning for predictive demand forecasting, or using robotic process automation to automate mundane work-flow tasks.
- However, AI and machine learning by itself is not sufficient. Supply chain organizations need to go from simply digitizing existing processes and workflows to reinventing the supply chain planning model to enable more flexible “sensing and pivoting.” For example, how would S&OP activate daily or even hourly for cross-functional decision trade-offs? What are the new feedback loops between production planning to demand forecasting?
- The discussion ended with a look at several steps to getting started: articulating a future vision of what S&OP model looks like; getting a handle on integrating disparate data sources for training and scaling AI and machine learning solutions, and developing a fail-fast minimum viable product mentality.
www.supplychain247.com
|